Introduction

Implementing artificial intelligence solutions is just the beginning of your journey. The real challenge lies in maintaining and scaling these systems as your business grows. Keeping your AI running efficiently requires ongoing effort, whether you’re working with pre-trained or custom models.
To make the most out of this powerful business tool, you must prioritize model performance, real-time decision-making, and scalable infrastructure. Here are some valuable insights to maximize your systems.
Continuous Monitoring and Feedback: The Key to AI Success
Integrating artificial intelligence or AI solutions into your business isn’t a one-time effort. It’s an ongoing process. Continuous monitoring ensures that your models deliver real-time insights and work as intended.
Leveraging MLOps Tools
AI solutions, particularly foundation models and machine learning models, can degrade over time due to changes in data patterns. Regular tracking and real-time monitoring tools help you maintain acceptable data quality. Machine learning operations (MLOps) platforms streamline model retraining and reduce manual interventions in AI maintenance.
Model retraining should become a regular part of your maintenance schedule. Some systems may need daily updates, while others might perform well with quarterly retraining. The frequency depends on how quickly the underlying data patterns change in your industry and the specific use case.
Establishing a Governance Team
A single team managing both model production and deployment may not be sufficient for large-scale AI solutions. Governance teams play a crucial role in ensuring accurate AI model performance and overseeing optimization processes.
Using Real-Time Monitoring Platforms
AI solutions should provide immediate insights to optimize downstream processes and activities across business processes. In financial services, for example, fraud detection models need real-time decision-making capabilities to respond to emerging threats. If you don’t track models continuously, security risks increase.
Real-time monitoring tools help you track models, analyze user experience, and adjust AI-based business operations accordingly. They’re particularly useful for customer service or lead generation efforts, which require constant fine-tuning to keep up with shifting consumer behavior.
Without proper monitoring, you risk outdated models that no longer align with customer preferences or business outcomes. Conversely, focusing on continuous monitoring and feedback ensures that AI solutions provide consistent, high-quality operations with minimal manual interventions.
Building a Scalable Infrastructure
Once your AI models are stable, the next step is scaling them. Growth requires advanced AI technology solutions, optimized business models, and strategic scalability efforts. However, businesses face major challenges when expanding AI solutions, including increased development time, additional technology investments, and behavioral impacts on existing workflows.
That’s why many businesses rely on external support. Washington-based companies, for example, benefit from specialized IT support Renton services that ensure scalable infrastructure and seamless AI implementation.
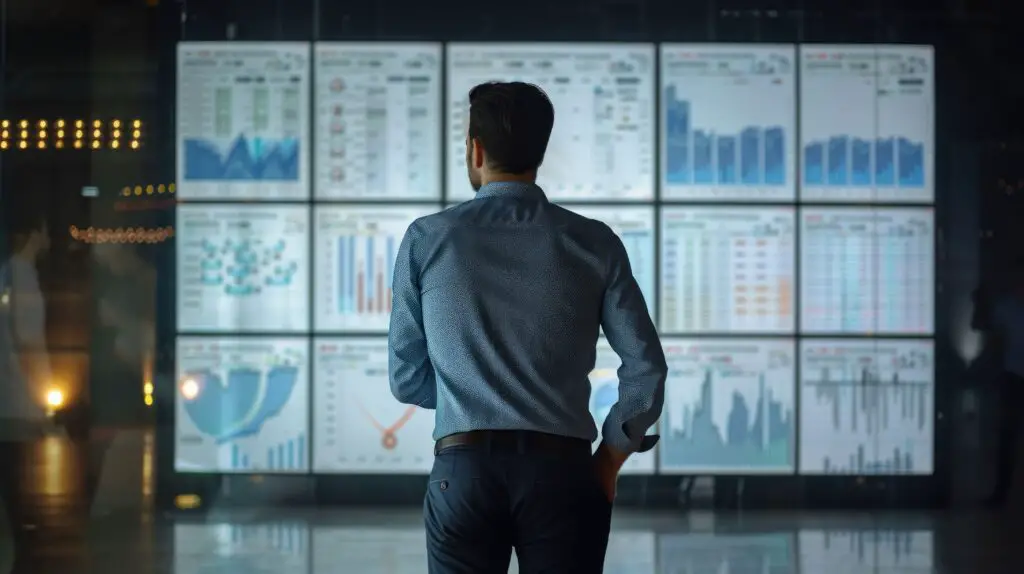
Strategies for Scaling AI
Scaling AI solutions successfully requires a combination of strong infrastructure, skilled personnel, and efficient processes. Without the right foundation, even the most advanced AI applications can struggle to adapt to growing demands or meet their business goals.
Adopting Cloud Technology
AI models at scale demand cloud platforms and cloud services for efficient processing. Cloud platforms offer significant advantages here, providing flexible resources that grow with your needs without requiring massive upfront investments in premise solutions.
Streamlining Model Training and Deployment
Scalability efforts require investment in cloud technology, software development, and IT support. Businesses must balance start-up investment with incremental modernization strategies. Implementing efficient model training processes, including pod model structures and modular app development, reduces development time.
Forming A Super Team
It’s in your best interest to build a dedicated center of excellence. This initiative brings together IT support, a science team, and an operations team to manage AI upkeep efficiently.
Optimizing AI-Related Skills Development
Expanding requires advanced AI skills across the science team and project team. Many companies lack the AI-related abilities needed to handle optimization processes and systems at scale. Upskilling your workforce in advanced AI skills and MLOps ensures smoother AI implementation, even if you rely on external providers.
Focusing on Quality Over Quantity
Many organizations struggle with integrating AI applications in business. Instead of deploying numerous models, focus on developing high-quality models that enhance product development and business outcomes.
Start by identifying where AI can deliver the most significant impact on business models and customer value management. Focus on quality over quantity—a few well-maintained systems often provide better results than dozens of poorly supported projects. For example, you can use AI analytics to improve supply chain efficiency if your business relies primarily on it.
Final Thoughts
Maintaining and scaling AI solutions demand attention to your entire infrastructure, organizational structure, and continuous improvement processes. Implementing scalable practices from the beginning helps you avoid many of the major challenges that organizations face when trying to grow their AI capabilities. Then, maximize the potential of artificial intelligence by tapping cloud services, automating model retraining, and integrating AI into daily operations.
The best tool for business leaders is having the right mindset. After all, successful AI scaling isn’t just about technology. It’s about aligning these powerful tools with your business objectives, customer preferences, and operational needs. With thoughtful planning and incremental modernization strategies, you can build systems that continue to deliver value long after the initial excitement of implementation fades.